CoCIS Researchers Design Models to Diagnose Malaria on a Smartphone using Artificial Intelligence (AI)
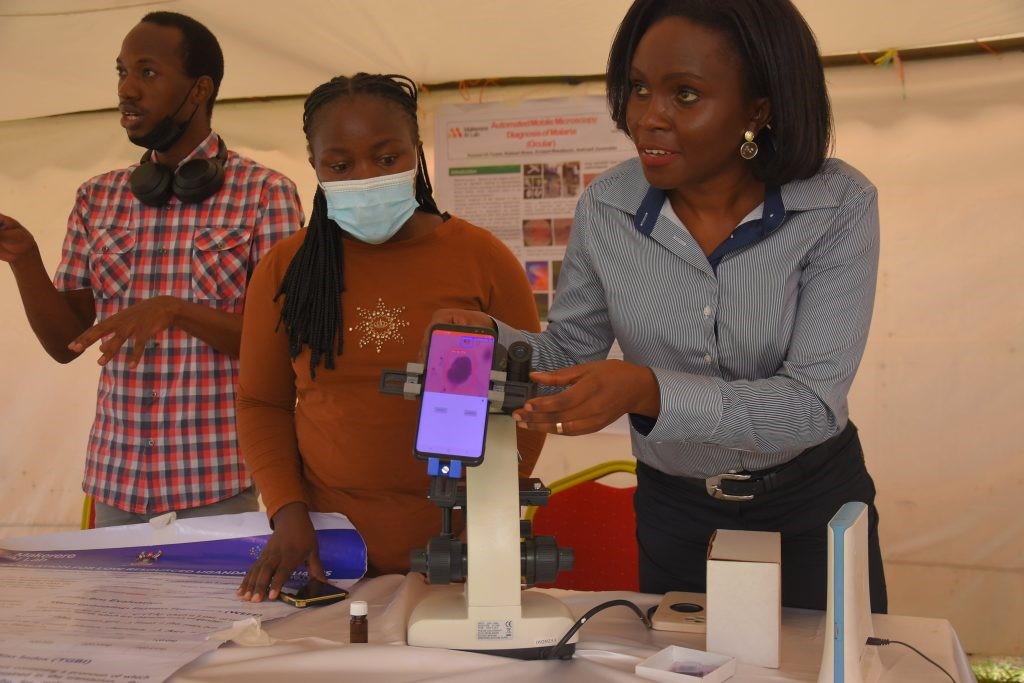
Dr. Rose Nakasi a researcher in the Automation of diagnosis of Malaria, specifically in thick blood smears using Computer Vision Models has been able to show how Artificial Intelligence can be used to diagnose malaria and improve the way the microscopy method is used in the diagnosis of malaria.
“In sub-Saharan Africa where the disease is highly endemic, there are few laboratory technicians in most of our health facilities to carry out the diagnosis of malaria, and some of the patients go with wrong and misdiagnosis sometimes based on symptoms which are not accurate.
So there is drug resistance and high mortalities. This research comes to help out is to augment microscopy using readily available equipment like the microscope but also available technology which is very common in the smartphone. Nakasi said
Nakasi was recently showcasing the technology during the Open Day organized by the College of Computing and Information Sciences (CoCIS) on 30th September 2022. The Open Day, Public Lecture, and Alumni Dinner were organized by a committee led by the College Grants Officer Ms. Dora Bampangana and Dr. John Ngubire under the theme, “The Future of Computing and Information Sciences/Services”.
“We want to be able to do a diagnosis on a smartphone using artificial intelligence. We are reducing the strain that microscopists face when they are looking out for pathogens in the microscopy samples but it is also going to give them confidence in the results that come out at the end of the day because we see the limitation of variation of judgment by microscopists”. Dr. Nakasi explained
The other motivation behind the research according to Dr. Nakasi is to ensure that microscopists abide by the Standard Operating Procedures barring them to view beyond the recommended number of slides per day. An expert in microscopy is recommended to look at only 20 slides in a day yet in real-life situations one can have more than 20 patients a day.
Dr. Nakasi stressed that there are so many patients that come in for diagnosis of malaria and that means if the SOPs are followed, most of them will go back without a clear diagnosis, and in the end, the disease will advance but also patients may take drugs, not for malaria.
“This research is to improve general health care, especially following the SDG 3 of Good Health and wellbeing of all humans. We believe that if we can be able to reduce or control malaria through a more accurate diagnostic method which we are proposing in our research using the computational method, this will shorten the time of diagnosis and improve the number of people diagnosed in a day and improve the general public health in highly endemic areas”. Dr. Nakasi explained.
She said the team is developing tools that can help achieve SDG3 to make it usable for remote areas, especially the mobile application where the innovation is put on a smartphone
Dr. Nakasi believes this mobile application will elevate the way the disease is diagnosed since a person can take his image on a smartphone and with this AI model on a smartphone, the analysis can be done offline without internet connectivity.
A team member that developed some components of automated microscopy Tusubira Francis Jeremy said the motivation for this work was to improve microscopy by automating some of the processes. Tusubira is a researcher in the AI Lab focused on computer vision as a machine learning engineer and soft engineering work.
Tusubira said, one of the challenges faced in microscopy includes a shortage of experts in microscopy even when the equipment is available because they can only look at 20 slides per day. He said the motivation for automating the process was to increase the throughput of samples that can be analyzed up to more than 100 slides.
“The first component of this research was analyzing the blood smear images which was done by adopting a custom 3D adaptor that is put on a microscope, then a phone is placed on that adapter to capture the image on the slide so that the analysis can be done on the image and not by looking directly into the eyepiece.
The second component of this work was to automate the detection of different artifacts within the images for malaria, there are models that have been developed to detect malaria trophocytes and white blood cells and these models have performed very well with high precision.
These models have been deployed and can be used on a phone, you take the picture on the phone but it also analyses the pictures on the phone or you can also upload the pictures for analyzing remotely on a web service”, Tusubira explained.
Tusubira said efforts are being made to deploy the models in the field, but this being Artificial Intelligence, health data, and dealing with people’s health, many things have to be taken into consideration.
“But the most important is that these models are not going to be let out there to run on their own, but there are simply decision support systems. There is always going to be an expert in the loop to validate what the models are doing”. He added.
The Research
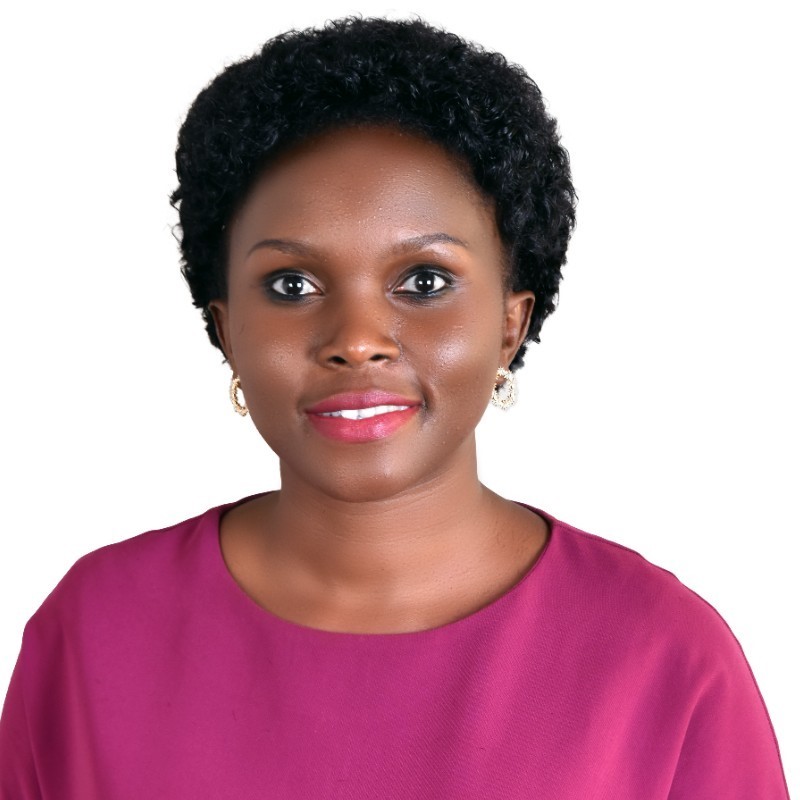
Brief BIOGRAPHY
Researcher, AI LAB, Makerere University Rose Nakasi, PhD is a Researcher of Computer Science at AI and Data Science Lab, Makerere University in Uganda as well as Chair for Topic Group AI-based detection of Malaria (TG-Malaria) at the ITU-Focus Group AI for Health (FGAI4H). Her research interests are in Artificial Intelligence and Data Science and particularly in the use of these for developing improved automated tools and techniques for diagnosis and prediction of Malaria in low resourced but highly endemic Countries. |
Dr. NAKASI Rose investigated how deep learning algorithms can be used for the automated detection of malaria and its parasitemia determination in microscopic thick blood smear images. Using an experimental design, the study revealed that by exploiting recent technological advances in 3D printing and deep learning to produce effective hardware and software respectively, a functioning point-of-care diagnosis system for malaria on this principle, capable of running on multiple microscopes and phone combinations can be produced.
A malaria parasite detection accuracy of over 98% as compared to conventional machine learning methods was achieved. This study contributes to the practical improved malaria diagnosis especially in highly endemic, but low-resource settings in Sub-Saharan Africa, where there are few trained lab experts. Further, the diagnostic solutions developed in this study could be adapted for the general microscopy disease diagnosis. The study was funded by SIDA and was supervised by Dr. Ernest Mwebaze and Dr. Aminah Zawedde.